Machine Learning Interpretability A Survey On Methods And Metrics
Interpretations Interpretability Trustworthiness and Beyond. Tists use of two existing interpretability tools the InterpretML implementation of GAMs and the SHAP Python package.
Figure 1 From Notes On Notebooks Is Jupyter The Bringer Of Jollity Semantic Scholar In 2021 Scholar Web Languages Computer Science
We conduct a contextual inquiry N11 and a survey N197 of data scientists to observe how they use interpretability tools to uncover common issues that arise when building and eval-uating ML models.
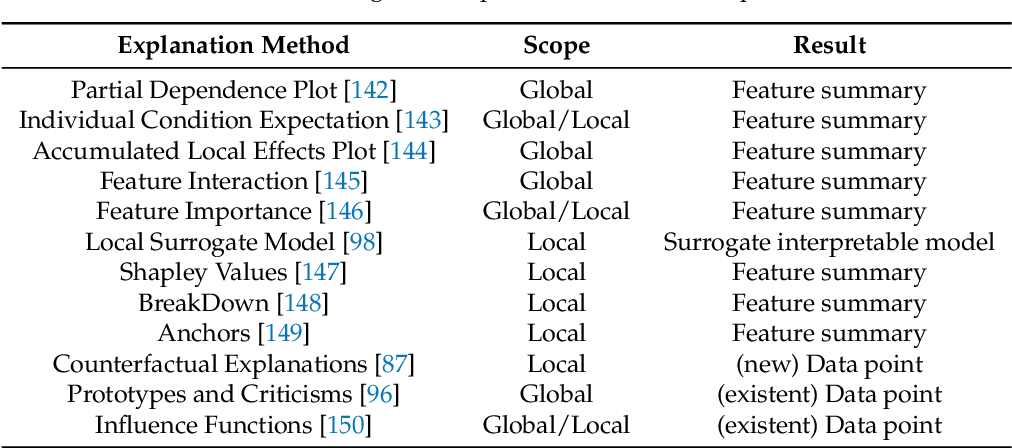
Machine learning interpretability a survey on methods and metrics. In addition this survey provides in-depth insights into the existing evaluation metrics for measuring interpretability which can help practitioners understand for what scenarios each evaluation metric is suitable. Deep neural networks have been well-known for their superb performance in handling various machine learning and artificial intelligence tasks. We present a 2D categorization of interpretable machine learning evaluation methods and show a mapping.
0 share. The identified properties of explainability are used as objectives that evaluation metrics should achieve. The authors also propose metrics for measuring the quality of an explanation.
The aim of this article is to provide a review of the current state of the research field on machine learning interpretability while focusing on the societal impact and on the developed methods and metrics. Our work supports the different evaluation goals in interpretable machine learning research by a thorough review of evaluation methodologies used in machine-explanation research across the fields of human-computer interaction visual analytics and machine learning. Interpretable Deep Learning.
The third group consist of advanced machine learning techniques such as SVM ensemble learning and deep learning methods. Keywords Interpratablity explainability causal inference counterfac-tuals machine learning 1. Kankanhalli Trends and The authors found the largest most central and well-studied network to be intelligence and ambient systems.
A Survey on Methods and Metrics. In this work we present a comprehensive survey on causal interpretable models from the aspects of the problems and methods. The survey found that the quantitative metrics for both model-based and example-based explanations are primarily used to evaluate the parsimonysimplicity of interpretability while the quantitative metrics for attribution-based explanations are primarily used to evaluate the soundness of fidelity of.
Furthermore a complete literature review is presented in order. In addition this survey pro-vides in-depth insights into the existing evaluation metrics for measuring interpretability which can help practitioners understand for what scenarios each evaluation metric is suit-able. This article surveys approaches for achieving interpretability in machine learning models and considers societal impacts of interpretability in sensitive audited and regulated deployments.
In addition this survey provides in-depth insights into the existing evaluation metrics for measuring interpretability which can help practitioners understand for what scenarios each evaluation metric is suitable. In this work we present a comprehensive survey on causal interpretable models from the aspects of the problems and methods. 1 When is it unethical to manipulate an explanation A.
As machine learning is being experimented with applied adopted in newer domains interpretability of ML models has become a hot topic both in academia industry. Understanding model interpretability to solve concrete problems is the first step towards successful Data Science projects. The authors note a lack of classical AI methods being applied to interpretability encouraging broader application of those methods to current research.
The problems and methods. 03192021 by Xuhong Li et al.
Pdf Machine Learning Interpretability A Survey On Methods And Metrics Semantic Scholar
Pdf Machine Learning Interpretability A Survey On Methods And Metrics Semantic Scholar
Pdf Machine Learning Interpretability A Survey On Methods And Metrics
Orchestrating The Development Lifecycle Of Machine Learning Based Iot Applications A Taxonomy And Survey Machine Learning Taxonomy Learning
Pdf Techniques For Interpretable Machine Learning Semantic Scholar
Figure 2 From Toward A Knowledge Based Personalised Recommender System For Mobile App Development Sem Recommender System Knowledge Management App Development
Pdf Machine Learning Interpretability A Survey On Methods And Metrics Semantic Scholar
Figure 5 From Semantic Integration In Big Data State Of The Art Semantic Scholar Big Data Scholar Web Technology
Techniques For Interpretable Machine Learning January 2020 Communications Of The Acm
Pdf Techniques For Interpretable Machine Learning Semantic Scholar
Table 5 From Machine Learning Interpretability A Survey On Methods And Metrics Semantic Scholar Machine Learning Learn Computer Science Surveys
Pdf Machine Learning Interpretability A Survey On Methods And Metrics
Pdf Machine Learning Interpretability A Survey On Methods And Metrics Semantic Scholar
Figure 9 From Design Research And Object Oriented Ontology Semantic Scholar Design Research Design Theory Human Centered Design
Pdf Machine Learning Interpretability A Survey On Methods And Metrics Semantic Scholar
Pdf Machine Learning Interpretability A Survey On Methods And Metrics Semantic Scholar
Interpretability Of Machine Learning Based Prediction Models In Healthcare Stiglic 2020 Wires Data Mining And Knowledge Discovery Nbsp Nbsp Wiley Online Library
Pdf Collaborative Cloud Computing Framework For Health Data With Open Source Technologies Semantic Schola Cloud Computing Sensors Technology Storage Server
Techniques For Interpretable Machine Learning January 2020 Communications Of The Acm
Post a Comment for "Machine Learning Interpretability A Survey On Methods And Metrics"